How to Catch Data Exfiltration with Machine Learning
Why is Detecting Data Exfiltration of Utmost Importance?
In today's landscape, there is an unprecedented surge in ransomware attacks and data breaches aimed at coercing businesses. Concurrently, the cybersecurity industry is confronted with numerous critical vulnerabilities within database software and corporate websites. These developments paint a grim picture of data exposure and unauthorized data removal that security leaders and their teams are contending with. This article sheds light on this challenge and elaborates on the advantages offered by Machine Learning algorithms and Network Detection & Response (NDR) methodologies.
Data exfiltration frequently marks the concluding phase of a cyberattack, representing the final chance to identify the breach before the data becomes public or is exploited for nefarious purposes like espionage. Nevertheless, data leakage isn't solely a result of cyberattacks; it can also occur due to human errors. While it's ideal to prevent data exfiltration through robust security measures, the increasing complexity and widespread distribution of infrastructures, combined with the integration of outdated devices, render prevention a challenging endeavour. In such situations, detection functions as our ultimate safeguard – indeed, it's better to detect it late than not at all.
Confronting the Difficulty of Detecting Data Exfiltration
Perpetrators can take advantage of multiple security vulnerabilities to collect and illicitly transfer data, utilizing protocols such as DNS, HTTP(S), FTP, and SMB. The MITRE ATT&CK framework delineates numerous patterns of data exfiltration attacks. Nevertheless, staying current with each protocol and infrastructure alteration is an imposing challenge, adding complexity to the pursuit of comprehensive security monitoring. What is required is a tailored analysis based on the volume of data, specific to devices or networks, with adjusted thresholds to enhance effectiveness.
This is where Network Detection & Response (NDR) technology comes into play. NDR powered by machine learning offers two significant capabilities:
- It enables practical monitoring of all relevant network communications, serving as the foundation for comprehensive data exfiltration monitoring. This includes not only interactions between internal and external systems but also internal communications. Some attacker groups transfer data directly outside, while others utilize dedicated internal exfiltration hosts.
- Machine learning algorithms play a pivotal role in adapting and learning context-specific thresholds for different devices and networks, which is crucial in the current diverse landscape of infrastructure.
Some of the key members of Exeon’s machine learning algorithms team, from left to right: Stefan Nyffenegger, Professional Services Engineer, David Gugelmann, CEO, and Markus Happe, CTO.
Unravelling Machine Learning for Data Exfiltration Detection
Before the advent of Machine Learning, the process involved manual configuration of thresholds specific to networks or clients. Consequently, an alert would be triggered if a device exceeded the predefined data threshold when communicating outside the network. However, the introduction of Machine Learning algorithms has ushered in several advantages for data exfiltration detection: 1. Acquiring knowledge of network traffic communication patterns and the upload/download behavior of clients and servers, providing a crucial foundation for identifying anomalies. 2. Establishing appropriate thresholds tailored to various clients, servers, and networks. Managing and defining these thresholds for each network or client group would otherwise be a laborious task. 3. Recognizing deviations in learned volume patterns, thereby detecting outliers and suspicious data transfers, whether they occur internally or involve exchanges between internal and external systems. 4. Utilizing scoring systems to quantify exceptional data points, establishing connections with other systems to assess the data, and creating notifications for detected irregularities.
Visualization: When the traffic volume surpasses a certain threshold, as determined by the learned profile, an alert will be triggered.
Machine Learning-Powered Network Detection & Response Comes to the Rescue
Network Detection & Response (NDR) solutions offer a holistic and insightful approach to identifying unusual network behaviors and sudden spikes in data transfer. By harnessing the capabilities of Machine Learning (ML), these solutions create a foundation for network communication patterns, enabling the rapid detection of anomalies, where it pertains to volume analysis or covert channels.
With this advanced and proactive approach, NDRs can identify the earliest indicators of intrusion, often well in advance of any data exfiltration occurrence.
The ExeonTrace platform: Data Volume Outlier Detection
A standout NDR solution known for its meticulous data volume monitoring, is ExeonTrace. Developed in Switzerland, this NDR system harnesses award-winning Machine Learning algorithms to passively scrutinize and assess real-time network traffic, pinpointing potential instances of risky or unauthorized data transfer. Notably, ExeonTrace seamlessly integrates with your current infrastructure, eliminating the need for additional hardware agents. The benefits of ExeonTrace go beyond just enhancing security; it also contributes to a deeper understanding of normal and unusual network activities, a pivotal aspect in fortifying and optimizing your overall security framework.
ML in Network Detection: Key Elements
In the contemporary digital landscape, network expansion and heightened vulnerabilities are constant challenges. Consequently, robust data exfiltration detection is imperative. However, given the intricacy of modern networks, manually establishing thresholds for outlier detection can be not only burdensome but also nearly impractical. By employing volume-based detection and monitoring traffic behaviors, one can spot data exfiltration by identifying deviations in data volume and upload/download traffic patterns. This underscores the potency of Machine Learning (ML) within Network Detection & Response (NDR) systems, automating the recognition of infrastructure-specific thresholds and anomalies.
Among these NDR solutions, ExeonTrace distinguishes itself by offering comprehensive network visibility, efficient anomaly detection, and a bolstered security posture. These attributes ensure that business operations can proceed securely and efficiently. To explore how ML-powered NDR can enhance data exfiltration detection and identify irregular network behaviors for your organization, we invite you to request a demonstration.
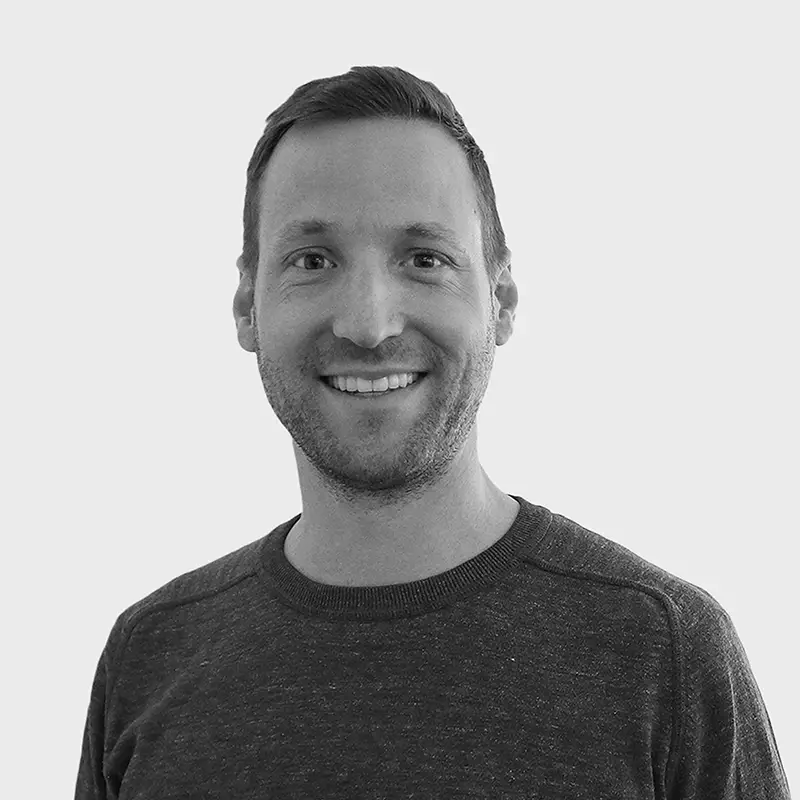
Author:
Andreas Hunkeler
Head of Professional Services
email:
andreas.hunkeler@exeon.com
Share:
Published on:
25.10.2023